Facultad de Informática
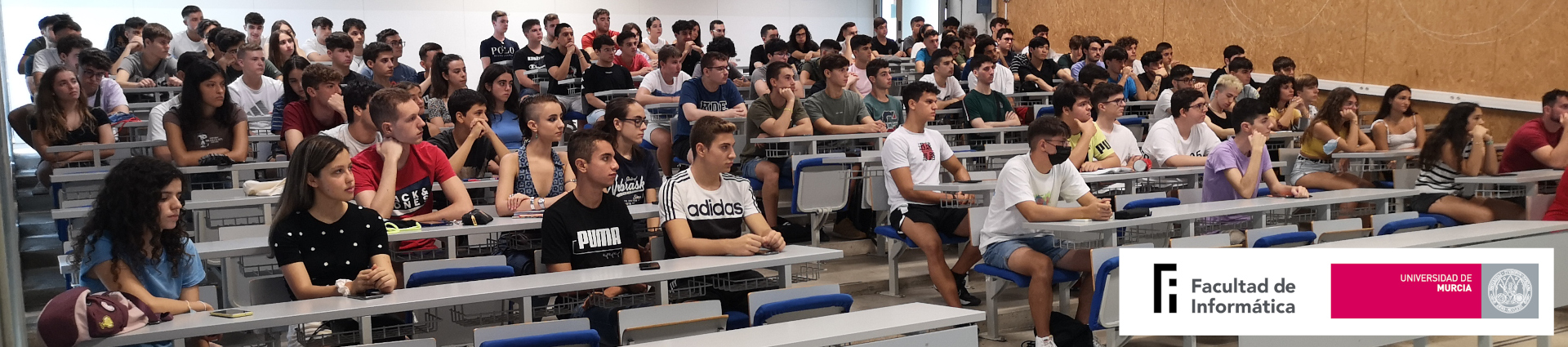
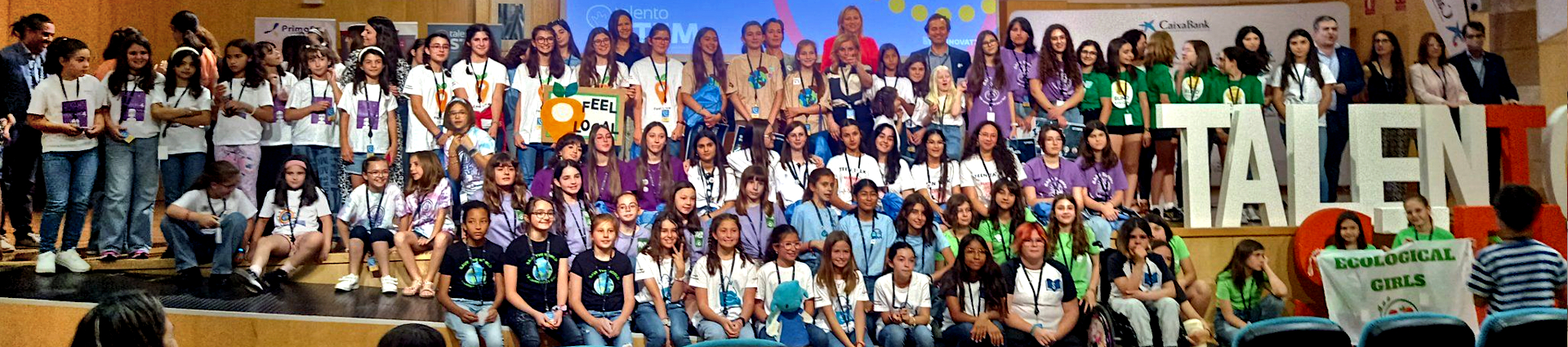
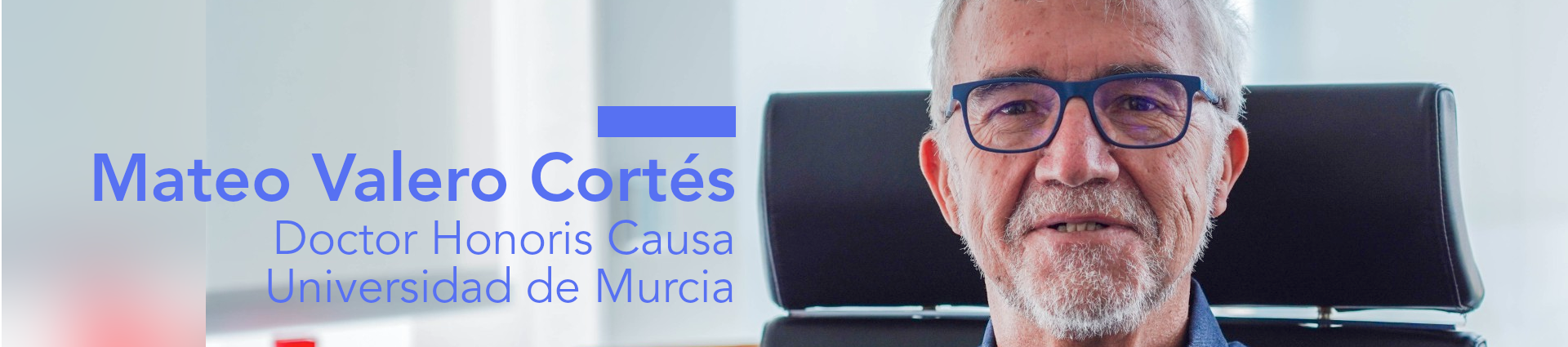
null Curso sobre optimización polinómica en la FIUM
Curso sobre optimización polinómica en la FIUM
La FIUM ofrece una actividad dirigida tanto a alumnos como a profesores de los grados de Ingeniería Informática y de Matemáticas. Se trata de un curso básico sobre Optimización Polinómica, impartido por los ponentes Jean Lasserre y Victor Magron, que son investigadores en el LAAS-CNRS (Laboratoire d'analyse et d'architecture des systèmes) de Toulouse. Ambos son reconocidos expertos internacionales en el área, con una amplia experiencia en investigación. En concreto Lasserre es coautor de más de 240 artículos de investigación y ha escrito, entre otros, el libro "An introduction to Polynomial and Semi-Algebraic Optimization".
Título: Introduction to the Moment-SOS hierarchy for polynomial optimization
Ponente: Jean B. Lasserre
Lugar: Aula A-01, Aulario Norte
Día y Hora: miércoles 18 mayo, 15:30-17:00
Resumen: In the first part of the talk one will briefly introduce the "Moment-SOS hierarchy" initially developed for solving polynomial optimization problems (i.e., finding the global minimum of a polynomial on a given semi-algebraic set "K" of R^n). It consists of replacing a usually intractable problem (whose description is algebraic) with a sequence of tractable convex optimization problems of increasing size. The resulting sequence of optimal values is monotone increasing and converges to the desired global optimum. Its rationale relies on results from real algebraic geometry (K-positivity certificates), the primal side, and "dual" results on the K-moment problem (the dual side in functional analysis). The list of potential applications of the Moment-SOS hierarchy in and outside optimization is almost endless and one will briefly describe some of them.
Título: Exploiting sparsity and symmetries in polynomial optimization
Ponente: Victor Magron
Lugar: Aula A-01, Aulario Norte
Día y Hora: miércoles 18 mayo, 17:30-19:00
Resumen: On the practical side, there is no free lunch and polynomial optimization methods usually encompass severe scalability issues. In the second part of this talk, we focus on tackling these issues. The underlying reason is that for optimization problems involving polynomials in n variables of degree at most 2d, the size of the matrices involved in the Moment-SOS hierarchy of convex relaxations is proportional to (n+2d) choose n. Fortunately, for many applications, we can look at the problem in the eyes and exploit the inherent data structure arising from the cost and constraints describing the problem, in particular sparsity or symmetries:
- correlative sparsity, occurring when there are few correlations between the variables of the input polynomials;
- term sparsity, occurring when there are a small number of terms involved in the input polynomials by comparison with the fully dense case;
- invariance of all input polynomials under the action of a subgroup of the general linear group.
In all cases, the properties on the original optimization problem induce specific sparsity/symmetry structures on the matrices arising in the convex relaxations. Such schemes have been used for important applications arising from various fields, including roundoff error bounds (computer arithmetic), robustness of deep networks (machine learning), entanglement (quantum information), AC optimal power-flow (energy networks), and sphere packing (discrete geometry).